ESTIMATING STROKE NUMBER AND CADENCE OF WHEELCHAIR PROPULSION USING PORTABLE SENSORS
ABSTRACT
Due to lower limb paralysis, individuals with spinal cord injury (SCI) rely extensively on their upper limbs for mobility and activities of daily living. While ample reports suggest that pain and injury is highly likely in long-term SCI, and overuse is blamed to be the cause (1, 2), the upper limb activities and the repetitiveness of these activities that occur on a daily basis is unclear. This paper presents a study where three tri-axis accelerometers placed on the upper arm, wrist, and under the wheelchair’s seat were used to estimate temporal parameters of wheelchair propulsion (e.g., stroke number and cadence). Thirteen participants with SCI were asked to push their chair equipped with a SmartWheel (SMW) on level and sloped surfaces while being videotaped. The estimated stroke number from each accelerometer and the SMW were compared with video observations. The estimated cadence from each accelerometer was compared with that from the SMW. Mean absolute errors (MAE) and mean absolute percentage of error (MAPE) were calculated between the estimated and criterion values. Intraclass correlation coefficients (ICC(3, 1)) were also calculated to assess the agreement. Results showed reasonable accuracy of the estimated temporal parameters using accelerometers, especially the one placed on the upper arm where the overall MAPE was 10.0% for stroke number, and 10.7% for cadence. These results suggested that accelerometers could be a viable option for monitoring upper limb movements of wheelchair propulsion in the natural environment of wheelchair users.
BACKGROUND
It is estimated that about 31% to 73% SCI has upper limb pain and injury (1). Previous studies have associated the prevalence of pain and injury among this group with wheelchair propulsion. Especially the cadence (i.e., the repetitiveness of the movement), and propulsion force were identified as two important factors for evaluating wheelchair propulsion (3, 4).
There are many monitoring tools and techniques that can be used to assess wheelchair propulsion by individuals with SCI. However, many of these tools such as motion capture systems and sensing wheels can only be used in clinical settings. With the recent advancement of sensors and miniature technologies, accelerometers emerge as a possible solution for measuring user movements in their natural environments without requiring observation and complicated setups.
Previous studies have used accelerometers to track gross mobility of wheelchair users and shown their validity in detecting mobility levels and wheelchair propulsion episodes. A study conducted by Coulter et al. used tri-axial accelerometers placed on the wheelchair’s wheels to distinguish the direction and duration of movement in manual wheelchair users. The study demonstrated excellent validity for wheel revolutions, absolute angle, and duration of movement (5). Postman et al. used six accelerometers to distinguish propulsion and hand biking from a range of activities of daily living among manual wheelchair users. The study demonstrated that wheelchair propulsion can be correctly detected by the use of accelerometers with a mean overestimation of 3.9 percent (6). A study conducted by Gendle et al. investigated participants’ heart rates and the distance traveled by placing accelerometers below the wheelchair seat of 12 basketball players. The results showed that activity counts and distances were significantly different between light and moderate effort (7).
Previous research on using accelerometers to track temporal parameters of wheelchair propulsion is very limited. A study conducted by Hiremath et al. investigated temporal parameters of wheelchair propulsion including stroke frequency, propulsion time, and recovery time based on hand acceleration collected via motion analysis system instead of portable sensors like accelerometers. The study showed high intraclass correlation coefficients for stroke time, propulsion time, and recovery time between the estimated and criterion measures (8). A study conducted by Turner et al. investigated the use of an accelerometer placed beneath the chair and a wheel mounted magnet to detect several wheelchair propulsion parameters including stroke number, cadence, distance, and speed. Ten manual wheelchair users were asked to propel their wheelchair at indoor and outdoor surfaces. Estimated parameters were compared with criterion values obtained from the OptiPush wheels. Results showed the average percentage of errors were -1.0% for stroke number and -1.7 % for Cadence (9).
The purpose of this study is to assess the validity of a tri-axis accelerometer placed at three locations (i.e., wrist, upper arm, on the wheelchair axle underneath the wheelchair seat) in estimating stroke number and cadence of wheelchair propulsion among manual wheelchair users with SCI.
METHODOLOGY
Experimental Protocol
The study was approved by the Institutional Review Board at the University of Pittsburgh. Subjects were recruited if they had SCI, were over 18 years old, and used a manual wheelchair for primary mobility. The exclusion criteria were: inability to tolerate sitting for more than two hours, and presence of upper limb pain that limits mobility. Subjects were required to answer a demographic survey and a Wheelchair Users Shoulder Pain Index (WUSPI) questionnaire. Participants were asked to propel their own wheelchairs on two surfaces including level surface (distance 33 meters) and sloped surface (distance 15 meters). A total of 24 level-surface trials performed at self-selected speed, low speed, and fast speed, and 12 sloped-surface trials at a self-selected speed were completed by each subject. For all propulsion trials, subjects’ wheels were replaced with a SMARTWheel (Three Rivers Holding Inc., Mesa AZ) with a sampling frequency of 240 Hz. at the dominant side and a dummy wheel at the other side to balance the weight of the SMARTWheel (SMW). In addition, monitoring devices consisting of three tri-axis accelerometers (Shimmer Research, Dublin, Ireland) and a custom wheel rotation data-logger were used to track upper limb movements of the subjects and the wheelchair motion. Two accelerometers with sampling frequency of 20 Hz. were place on the upper arm and wrist respectively. A third accelerometer with sampling frequency of 60 Hz. was placed on the axle beneath the seat. All trials were videotaped using a hand-held digital video recorder. The video recorder and all the devices used were synchronized against the same clock.
Data Analysis
Custom MATLAB® (Version 7.11.0 R2010b, The Mathworks, Inc USA) was used to process the data. Two investigators observed the videos and independently counted the stroke number for each trial. Videos were re- examined when there was a discrepancy between the two investigators. The videos recorded served as the criterion measures for stroke number. The SMW was the criterion measure for the estimated cadence.
Data from the wheel rotation data-logger was converted to the wheel speed, which was used to segment the acceleration data for each trial. Data from the accelerometers was filtered using an 8th-order Butterworth low-pass filter with a variable cutoff frequency defined through the fundamental frequency. To obtain the stroke number from the arm and wrist accelerometers, the resultant accelerations (the vector sum of three directions) were used. For the seat accelerometer, only the longitudinal component (parallel to the propulsion direction) was used. An algorithm was developed to extract the stroke number and cadence for each trial. The algorithm first calculates a threshold defined as the mean acceleration plus one standard deviation. The stroke numbers are then counted as the number of acceleration peaks over the established threshold. Cadence is obtained as the mean inverse propulsion time of each stroke. Propulsion time was defined as the time between two consecutive local minimum accelerations. The stroke number and cadence from the SMW were directly obtained from the SMW software.
The estimated stroke number from each accelerometer and the SMW were compared with video observations. The estimated cadence from each accelerometer was compared with that from the SMW. Mean absolute errors (MAE) and mean absolute percentage errors (MAPE) between the estimated and criterion values were calculated. In addition, the Intraclass correlation coefficient (ICC(3, 1)) 2-way mixed model, consistency, single measure) with 95% confidence interval (CI) was used to assess the agreements. Bland-Altman plots were also performed to provide a visual analysis of the agreements. All statistical analysis was performed using SPSS software (ver. 18.0, SPSS Inc., Chicago, IL, USA).
RESULTS
Thirteen participants including three females and 10 males with SCI and an average age of 38±14 years were tested in the study. The number of years that subjects have been using a manual wheelchair was 15±9 years and all subjects use their wheelchair over six hours a day. The WUSPI questionnaire measures shoulder pain based on 15 questions with each question on a 10cm visual analogue scale, resulting in a total score range from 0cm (no pain) to 150cm (extreme pain). The mean score among participants was 5.2 ± 8.0 cm. The top three common activities with the highest pain score were: pushing up ramps, lifting objects down from an overhead shelf, and sleeping.
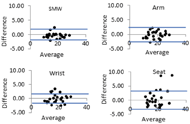
Table 1 and 2 show the mean and standard deviation (SD) of the criterion and estimated temporal parameters. Table 3 and 4 show the mean absolute error (MAE) and the mean absolute percentage of error (MAPE) between the criterion stroke number from video observations and the estimated stroke number from each accelerometer and the SMW. Table 5 and 6 show the MAE and MAPE between the criterion cadence from the SMW and the estimated cadence from each accelerometer. All variables were calculated for the level trials (LV), the up sloped trials (US), and the overall trials (OA). Table 7 shows the ICC (3, 1), upper and lower bounds within a 95% CI, and p-values between criterion and the estimated values. Figure 1 and 2 show the Bland-Altman plots of the criterion and estimated temporal parameters. Lines are placed to indicate upper and lower bounds defined as ± 2 SD.
DISCUSSION
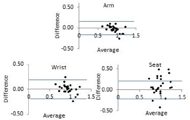
This study provides insight into the usage of portable devices (e.g., 3-axis accelerometer and wheel rotation data-logger) to determine upper limb movements for wheelchair propulsion. The relatively small errors (Table 3-6) and high intraclass correlation coefficients (Table 7) indicate the validity of this technique in measuring temporal parameters of wheelchair propulsion in not only clinical settings but also in the natural environment.
In terms of estimating the stroke number, the SMW showed the highest accuracy with an overall MAPE of 6.2%. This small error could be due the fact that some participants occasionally pushed their chairs on the wheel instead of on the instrumented handrim which was designed to sense the propulsion forces and determine the stroke number. The arm and wrist accelerometer showed relatively high accuracy with a MAPE of 10.0% and 14.1% respectively. Difference in accuracy between the arm and wrist accelerometers suggested that the upper arm might be a better location for detecting the stroke number. The wrist accelerometer was more sensitive to arm movements, possibly leading to the increased error. The seat accelerometer showed the lowest accuracy with a MAPE of 20.2%. This high MAPE could be related to the way it was placed. The seat accelerometer was attached to the wheelchair axel beneath the seat instead of directly under the seat which could be more sensitive to the wheelchair movements responding to each push.
Video | SMW | Arm | Wrist | Seat | |
---|---|---|---|---|---|
LV | 21±5 | 20±5 | 22±4 | 22±4 | 22±6 |
US | 16±4 | 16±4 | 15±4 | 15±4 | 16±5 |
OA | 18±5 | 18±5 | 18±5 | 18±5 | 19±6 |
SMW | Arm | Wrist | Seat | |
---|---|---|---|---|
LV | .88 ±.15 | .86±.10 | .89±.09 | 1.0±.18 |
US | 1.0±.16 | .96±.13 | 1.0±.13 | 1.0±.23 |
OA | .94±.17 | .91±.12 | .95±.13 | 1.0±.20 |
LV | 1.1±.77 | 2.0±1.3 | LV 2.9±1.9 | 3.9±2.6 |
---|---|---|---|---|
US | 1.0±.60 | 1.4±0.6 | 2.0±0.8 | 3.1±2.3 |
OA | 1.0±.68 | 1.7±1.0 | 2.5±1.5 | 3.5±2.4 |
SMW | Arm | Wrist | Seat | |
---|---|---|---|---|
LV | 5.4±4.7 | 10.6±7.7 | 15.1±10.6 | 19.8±11.8 |
US | 6.8±4.5 | 9.4±3.9 | 13.1± 5.8 | 20.6±14.4 |
OA | 6.2±4.6 | 10.0±6.0 | 14.1± 8.5 | 20.2±12.9 |
Arm |
Wrist |
Seat |
|
---|---|---|---|
LV | .13±.09 | .16±.10 | .33±.19 |
US | .08±.05 | .11±.08 | .24±.15 |
OA | .10±.07 | .14±09 | .28±.17 |
Arm
|
Wrist | Seat |
|
---|---|---|---|
LV | 14.0±9.1 | 19.1±12.0 | 41.8±26.7 |
US | 7.5± 3.8 | 11.5±10.0 | 23.1±12.7 |
OA | 10.7±7.6 | 15.3±11.5 | 32.2±22.4 |
ICC | 95% CI | p-value | ||
---|---|---|---|---|
Stroke Number | SMW | 0.981 | 0.937~0.987 | 0.000 |
Arm | 0.971 | 0.937~0.987 | 0.000 | |
Wrist | 0.949 | 0.889~0.977 | 0.000 | |
Seat | 0.856 | 0.708~0.932 | 0.000 | |
Cadence | Arm | 0.825 | 0.644~0.918 | 0.000 |
Wrist | 0.799 | 0.600~0.905 | 0.000 | |
Seat | 0.674 | 0.081~0.581 | 0.050 |
Regarding cadence estimation, arm and wrist accelerometers performed better with a MAPE of 10.7% and 15.3%, respectively than the seat accelerometer with a MAPE of 32.2%. These results suggested that placing the accelerometer on the axle beneath the seat may not be feasible to accurately detect cadence. Compared with the stroke number estimation, cadence estimation was less accurate, which could be due to the estimation of the total cycle time (push and recovery phases). The estimation algorithm based on the accelerometer signals was able to identify the push phase more accurately, but unable to accurately determine the end of recovery phases, leading to the inaccuracy when estimating the cycle time.
Turner et al. placed an accelerometer right beneath the wheelchair seat to estimate stroke number and cadence among 10 manual wheelchair users. Unfortunately, the data analysis results were not described in details. The study only reported an average percent error (i.e., -1.0% for stroke number and -1.7 % for cadence) instead of the MAPE averaged by each trial of each subject. An average percent error only indicates the estimation bias and may not be sufficient to show the estimation accuracy, as the positive and negative estimation errors from the trials may cancel each other, resulting in smaller overall errors.
Previous studies have attempted to use activity monitors to detect gross mobility levels in terms of traveling distance, speed (5,7,8,10) and wheelchair propulsion episodes (6). This study has shown that combining the upper limb accelerometer and wheel rotation datalogger has the potential to not only detect gross mobility levels of wheelchair users, but to quantify the quality of upper limb movements in terms of its repetitiveness. Considering the negative impact that upper limb pain and injuries have among manual wheelchair users with SCI, it is necessary to monitor and understand how the use of upper limbs during wheelchair propulsion and other ADLs are related to such pain and injury. A Clinical Practice Guideline on the Preservation of Upper Limb Function Following Spinal Cord Injury mentions the importance of reducing the frequency of repetitive upper limb tasks (9). This study could result in a potential tool that can monitor the actual usage of upper limbs in terms of the repetitiveness during propulsion, and contribute to the preservation of upper limb functions among manual wheelchair users with SCI.
Future work will be conducted to look into the relationship between acceleration features and propulsion forces, and use other type of portable sensors to obtain more kinematic parameters such as joint angles.
REFERENCES
- Rice, I., Gagnon, D., Gallagher, J., Boninger, M. (2010). Hand Rim Wheelchair Propulsion Training Using Biomechanical Real-Time Visual Feedback Based on Motor Learning Theory Principles. Journal of Spinal Cord Medicine, 33(1), 33-42.
- Pentland WE, Twomey LT. (1994) Upper limb function in persons with long term paraplegia and implications for independence: Part II. Paraplegia. 1994;32(4):219–24.
- Boninger, M.L et al., (2005) Pushrim biomechanics and injury prevention in spinal cord injury: recommendations based on CULP-SCI investigations. Journal of Rehabilitation Research & Development, 42, pp. 9-19
- Preserving Upper Limb Function in Spinal Cord Injury: A Clinical Practice Guideline for Health-Care Professionals. 2005. Consortium for Spinal Cord Medicine. Spinal Cord Medicine, Clinical Practice Guideline.
- Coulter, E H P M Dall, L Rochester, J P Hasler and M H Granat (2011) Development and validation of a physical activity monitor for use on a wheelchair. Spinal Cord 49, 445- 450
- Postma, K., et al. (2005) Validity of the detection of wheelchair propulsion as measured with an Activity Monitor in patients with spinal cord injury. Spinal Cord (2005) 43, 550–557
- Gendle, S., Richardson, M., Leeper, J., Hardin, B., Green, M., Bishop, A., (2010) Wheelchair-mounted accelerometers for measurement of physical activity. Disability and Rehabilitation: Assistive Technology 2010 DOI: 10.3109/17483107.2011 49, 445-450
- Hiremath, S., Ding, D., Koontz, A., (2008) Estimating Temporal Parameters of Wheelchair propulsion based on hand Acceleration", RESNA Annual Conference.
- Turner, J., Emfinger, W., (2011) The PushTracker, an Interactive Activity Monitor for Manual Wheelchair Users. RESNA Student Design Competition
- Tolerico, M. L. et al (2007). Assessing mobility characteristics and activity levels of manual wheelchair users. Journal of Rehabilitation Research & Development, 44(4), 561-571.
ACKNOWLEDGEMENTS
This research was supported by RERC SCI Research Center(#H133E070024). We gratefully acknowledge the contributions of Shivayogi Hiremath and Annmarie Kelleher.