Leia C. Shum1, Bulmaro A. Valdés2, H.F. Machiel Van der Loos1
1The University of British Columbia, 2Simon Fraser University
INTRODUCTION
For youth affected with motor disabilities, traditional repetitive task motor rehabilitation can be tedious, and the lack of stimulation and motivation can slow progress towards rehabilitative goals [1]. With the increased availability of low-cost commercial gaming devices, researchers have investigated their potential as alternative tools for at-home rehabilitation [2]. Using devices such as the Nintendo Wii [3] and Microsoft Kinect [4,5], measurable improvements in upper limb performance have been measured. With the emergence of commercially available immersive virtual reality (VR) technology with motion-tracked controls, further exploration into engagement in exergaming is required [6].
With the use of accurate VR head-mounted displays (HMDs), the user’s view of the real world can be hidden, which can allow non-veridical and/or augmented visual feedback to be presented in a convincing manner. This capability allows for the exploration of concepts such as error augmentation (EA), in which real-time movement feedback provided without a physical constraint to the non-paretic side is adjusted based on the amount of movement “error”, as specified by the user’s rehabilitative goals. There have been limited rehabilitative error augmentation studies so far that use completely immersive virtual reality technology [7], despite prior evidence that unimanual error augmentation in 2D environments can increase the quality of upper limb rehabilitation [8]. Additionally, prior studies do not take advantage of mirrored movement or bimanual task training to provide inherent visual comparisons between asymmetric motor capabilities in persons with hemiplegia. An investigation of the use of gaming and motion-controlled technology with 3D VR environments for upper limb rehabilitation is needed to analyse the effectiveness of visual feedback to compare the weaker and stronger side during a symmetric bimanual reaching task.
The purposes of this paper are to: (1) outline an implementation of a virtual environment with visual EA using commercially available gaming technology to test if a decrease in the amount of asymmetry between the stronger and weaker limb during a bilateral reaching task can be observed and (2) disseminate early results from analysis of kinematic data from two participants with hemiplegic motor dysfunction as a result of Cerebral Palsy (CP).
METHODS
System Description
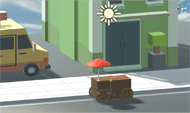
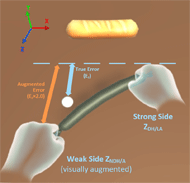
The 3D virtual environment (Fig. 1, left) was developed in Unity 3D 5.0 (Unity Technologies, San Francisco, CA, USA). The participants’ position, orientation, and visual representation of their hands were rendered using the OVRAvatar package to interface with the Oculus Rift (Oculus VR, LLC., Menlo Park, CA, USA) system including the HMD Headset, Oculus Touch controller pair, and 2 Oculus Sensors used to facilitate the VR environment in a 2x2m physical space.
Study Procedure
In a single-session, study participants trained with and without visual EA delivered in a randomized order. The participants were blinded to the order and were only made aware of augmentation effects at the end of the session. The full study session was broken into two sets of baseline (5 reaches), training (60 reaches) and evaluation (5 reaches) trials and a mandatory 5-minute break in between during which participants were instruct-ed to remove the HMD. Three reaches were performed at the start of the session to familiarize participants with the task and setup. During this stage, no visual augmentation was applied. In all trials, participants were asked to perform a reaching exercise that required them to pick up and place a virtual object at a specified location with both hands simultaneously (i.e., placing a ‘hotdog’ in a ‘bun’, pushing a towel across a table, putting ingredients in a sushi roll). The task was designed to function without the use of buttons or triggers for limited grasp function. An example of the reaching task is shown in Fig. 1 (right), where the goal is to move the cylindrical object to the oblong end goal object. These tasks were chosen to simulate two-handed activities of daily living such as carrying trays, large containers, and bulky objects. As the reaching movement was performed, the for-ward reaching distance between the hands (Ez = ZDH/LA – ZNDH/A) was calculated and used to augment the virtually rendered position in the forward reaching direction (z-axis) of the weaker side to visually amplify the symmetrical error by a factor of 2. This 2x factor originated from unimanual EA studies [9,10], in which this amount of amplification was shown to be larger than just barely noticeable, but not so large as to overly affect achieving the task goal.
Participants
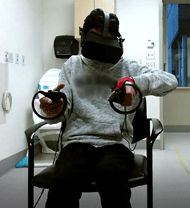
Participants were recruited through clinical contact at the BC Children’s Hospital (BCCH) Outpatient OT Department where each 1-2 hour exercise training session was carried out to test the effect of EA in the VR environment. Research Ethics Board approval for this study was obtained from the University of British Columbia, as was approval for utilization of BCCH resources, along with informed consent from all participants. To describe the participants’ upper limb motor ability with validated clinical scales, a short assessment prior to the study session was conducted to determine their Manual Ability Classification Scale (MACS) [11] and Bimanual Fine Motor Function (BFMF) [12] scores.
Participant 1 (14 years old, Fig. 2) was right handed with left hemiparetic cerebral palsy due to perinatal intraventricular hemorrhage and scored MACS II and BFMF II in upper limb motor ability. Participant 2 (19 years old) was left-hand dominant with right hemiparetic CP due to neonatal intra-parenchymal hemorrhage and scored MACS I and BFMF II. Some asymmetry was visible in both participants’ active ROM for shoulder flexion and extension, and both had limited grasp control in their weaker upper limb, allowing them to hold the controller but not activate any of the buttons or triggers.
Data Analysis
Kinematic data was collected from the Oculus devices at a variable sampling rate of 50-100Hz. Custom-developed MATLAB 2018b (MathWorks, Natick, USA) scripts were used to resample the data at a fixed frequency of 30Hz. A low-pass Butterworth filter with a cut-off frequency of 6Hz was used to smooth the reaching velocity profile of each trial, as is commonly done in upper limb motion studies [13]. The starting point was calculated as the timepoint when 10% of the maximum velocity was reached, and the end of the task occurred when both ends of the virtual object touched the goal region.
RESULTS AND DISCUSSION
For each participant, the (baseline) first 5 trials, and (evaluation) last 5 trials of the two training sets were used to calculate an average root-mean-squared asymmetry value for the distance between weaker and stronger side reaching position throughout the trial. In Table 1, the percent change for each training set was calculated as the error difference between baseline and evaluation, divided by the average baseline value such that a negative (bolded) percent change represents improvement from baseline symmetry, and a positive change represents a percent increase in symmetry error. A lower value in both raw error values and in percent change signify better symmetry and lower error produced.
Training set | Participant 1 - EA in second set (Set B) | Participant 2 - EA in first set (Set A) | ||||
---|---|---|---|---|---|---|
Baseline | Evaluation | % Change | Baseline | Evaluation | % Change | |
Without EA | 2.75 ± 1.85 | 5.15 ± 1.37 | 87.4% | 3.47 ± 0.73 | 5.95 ± 1.26 | 59.3% |
With visual EA | 2.91 ± 0.52 | 2.43 ± 1.13 | -16.2% | 3.69 ± 0.92 | 2.34 ± 0.54 | -36.6% |
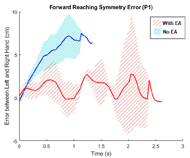
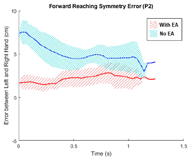
For P1 (Fig.3, left), while the average difference between left and right during forward reaching decreased for the set with EA applied, there are more ‘corrective’ movements and the average reach takes longer. For P2 (Fig.3, right), there are fewer corrective peaks in error but still an overall decrease in asymmetry compared to the No EA condition. P2 completed trials in both sets in approximately the same length of time as P1 without EA. It was observed from video recording that more involuntary wrist flexion occurred towards the end of the session. Due to the wide tolerance of object collision used to make it easier to pick up virtual objects, this allowed the starting point to be more asymmetrical for P2 when no EA was applied, increasing the average trial symmetry error.
It is important to note that when asked after completing the session, P1 noted a difference between the EA and no EA sets, while P2 did not. This may be a contributing factor to the difference in error profiles over the reaches with and without EA between the two participants, in addition to differences in initial motor ability. Previous results from sessions with typically developing teens and young adults of the same age range corroborate a greater improvement in bilateral forward reaching symmetry when visual EA is applied but found no significant difference in improvement based on the order of presenting the two training sets.
CONCLUSIONS
Visual error augmentation in immersive VR was found to decrease asymmetry in bimanual forward reaching for two persons with hemiplegic CP. Advances in commercially available gaming technology could be harnessed to supplement exergaming technology with increased rehabilitative gains and enriched real-time feedback. Results from this study will be used in conjunction with a larger sample size of participants with hemiplegia to further explore if there are significant differences in motor performance when using visual error augmentation. Comparison to motor adaptation in typically developing participants is suggested for future work. In addition, the evaluation of a possible increase in motor compensation techniques such as elbow elevation or trunk compensation is required.
REFERENCES
[1] Bryanton C, Bossé J, Brien M, Mclean J, Mccormick A & Sviestrup H. (2006). Feasibility, Motivation, and Selective Motor Control: Virtual Reality Compared to Conventional Home Exercise in Children with Cerebral Palsy. CyberPsychology & Behaviour, 9(2), 123–128.
[2] Valdés BA, Glegg SMN, Lambert-Shirzad N, Schneider AN, Marr J, Bernard R, …Van der Loos HFM. (2018). Application of Commercial Games for Home-Based Rehabilitation for People with Hemiparesis: Challenges and Lessons Learned. Games for Health Journal, 7(3), 197–207. https://doi.org/10.1089/g4h.2017.0137
[3] Winkels DGM, Kottink AIR, Temmink RAJ, Nijlant JMM & Buurke JH. (2013). WiiTM-habilitation of upper extremity function in children with Cerebral Palsy. An explorative study. Developmental Neurorehabilitation, 16(1), 44–51. https://doi.org/10.3109/17518423.2012.713401
[4] Chang Y-J, Han W-Y & Tsai Y-C. (2013). A Kinect-based upper limb rehabilitation system to assist people with cerebral palsy. Research in Developmental Disabilities, 34(11), 3654–3659. https://doi.org/10.1016/J.RIDD.2013.08.021
[5] Mousavi Hondori H & Khademi M. (2014). A Review on Technical and Clinical Impact of Microsoft Kinect on Physical Therapy and Rehabilitation. Journal of Medical Engineering, 2014, 1–16. https://doi.org/10.1155/2014/846514
[6] Levin MF, Weiss PL & Keshner EA. (2015). Emergence of virtual reality as a tool for upper limb rehabilitation: incorporation of motor control and motor learning principles. Physical Therapy, 95(3), 415–25. https://doi.org/10.2522/ptj.20130579
[7] Palacios-Navarro G, Albiol-Pére S & García-Magariño García I. (2016). Effects of sensory cueing in virtual motor rehabilitation. A review. Journal of Biomedical Informatics, 60, 49–57. https://doi.org/10.1016/J.JBI.2016.01.006
[8]Majeed YA, Abdollahi F, Awadalla S & Patton J. (2015). Multivariate outcomes in a three week bimanual self-telerehabilitation with error augmentation post-stroke. In 2015 37th Annual International Conference of the IEEE Engineering in Medicine and Biology Society (EMBC) (pp. 1425–1431). IEEE. https://doi.org/10.1109/EMBC.2015.7318637
[9] Wei Y, Bajaj P, Scheidt R & Patton J. (2005). Visual Error Augmentation for Enhancing Motor Learning and Rehabilitative Relearning. In IEEE International Conference on Rehabilitation Robotics ICORR (pp. 505–510).
[10] Shirzad N & Van der Loos HFM. (2012). Error amplification to promote motor learning and motivation in therapy robotics. In 2012 Annual International Conference of the IEEE Engineering in Medicine and Biology Society (pp. 3907–3910). IEEE. https://doi.org/10.1109/EMBC.2012.6346821
[11] Eliasson A-C, Ot P, Krumlinde-Sundholm L, Rösblad B, Pt P, Beckung E, … Frcp M. (2006). The Manual Ability Classification System (MACS) for children with cerebral palsy: scale development and evidence of validity and reliability. Developmental Medicine & Child Neurology, 48(7), 549–554. Retrieved from https://onlinelibrary.wiley.com/doi/pdf/10.1111/j.1469-8749.2006.tb01313.x
[12]Elvrum A-KG, Beckung E, Sæther R, Lydersen S, Vik T & Himmelmann K. (2017). Bimanual Capacity of Children With Cerebral Palsy: Intra- and Interrater Reliability of a Revised Edition of the Bimanual Fine Motor Function Classification. Physical & Occupational Therapy In Pediatrics, 37(3), 239–251. https://doi.org/10.1080/01942638.2016.1185507
[13] Enoka RM. (2008). Neuromechanics of human movement. Human Kinetics.
ACKNOWLEDGEMENTS
We would like to acknowledge Daphne O’Young (BC Children’s Hospital, Vancouver, CA), Candy Lin, the CARIS Lab at UBC, and the study participants. The work was completed through financial support from the Kids Brain Health Network (KBHN).