Kalai Tsang1,2, Zijian Huang1,2, Khara James1,2, Dan Ding1,2
1Department of Rehabilitation Sciences and Technology, School of Health and Rehabilitation Sciences,
University of Pittsburgh, PA., 2Human Engineering Research Laboratories, Department of Veteran Affairs Pittsburgh Healthcare System, Pittsburgh, PA
INTRODUCTION
There are 2.8 million manual wheelchair users (MWUs) in the United States [1]. Despite having clear physical activity (PA) guidelines, half of MWUs still lead a sedentary lifestyle due to many barriers they face such as lack of accessible facilities, transportation, and knowledge of PA [2]-[3]. Evidence shows that insufficient PA is associated with cardiorespiratory issues, diminished self-concept or other health conditions [4]; while sufficient PA is linked to improved functional capacity and reduced risks of secondary conditions [5]. With the proliferation of smartphones and wearable devices, mHealth based applications are becoming increasingly popular to address health promotion in the general population. However, similar applications are not widely available and tested among MWUs. There is a lack of wearable devices and mHealth applications specifically designed for this population. Only a few studies addressed PA promotion in MWUs using tele-based interventions. Arbour-Nicitopoulos et al. evaluated the effectiveness of a PA telephone service for 53 Canadians with spinal cord injury (SCI) for a 6-month intervention, and found that there was an increase in the percentage of participants who were regularly active at baseline (35%) vs 4-month (48%) and 6-month (52%) [6]. Hiremath et al. have an ongoing project evaluating a mHealth PA intervention system along with wearable devices for Individuals with SCI [7].
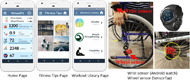
METHOD
This study was approved by the Institutional Review Board at the University of Pittsburgh. A convenient sample of MWUs were recruited. The inclusion criteria were: 1) between 18- to 65-year-old, 2) at least one-year post injury (if acquired) and medically stable, 3) used a manual wheelchair as their primary means of mobility, 4) lived in a community, and 5) had experience using a smartphone. MWUs who were 1) had any active pelvic or thigh wounds, 2) had contraindications to PA or exercises, and 3) pregnant (based on self-report) were excluded from this study.
This study contained a pre-trial lab visit, a 1-week home trial, and a post-trial lab visit. During the pre-trial lab visit, participants completed a questionnaire including demographics, wheelchair information, exercise/dietary habits, the stages of change of PA [8], and an Exercise Self Efficacy Scale (ESES) [9]. The study investigator first briefly explained how to use WheelFit including its accompanying devices and the app. Participants were then asked to wear the devices and explored the app on their own until they felt comfortable. They were also asked to fill out a questionnaire on their first impressions and initial interests of the app to check user acceptance of the concept of WheelFit before extensive experience using the app. Next, participants were asked to complete 6 tasks to ensure they understood how to use WheelFit app prior to the home trial. These tasks were considered completed when the participants indicated that they were done, asked for help, or gave up. Upon the end of the first visit, a take-home package including the two wearable devices and a study smartphone (a Huawei Mate 9 Lite phone or a Samsung Galaxy S6 phone) with the WheelFit app installed, and a troubleshooting guide was given to each participant. Before they left home with the package, participants were instructed to set their own fitness goal and encouraged to use the app daily during the 1-week home trial. During the 1-week home trial, participants were asked to voice record any minor issues they experienced when using the app (e.g. app crash and lag). They were also asked to contact the study investigator for help if they experienced any major technical difficulties (e.g. app is not opening, sensor disconnections). If the problem remained unsolved, the home trial would be terminated, and participants would be given a chance to restart the trial after problems were resolved. After completion of the home trial, participants visited the lab to return all devices and were asked to complete two post-home trial questionnaires to evaluate the overall functionality of the app as well as its content and design. A semi-structured interview was conducted to further collect detailed feedback on user experience using WheelFit. The interview was voice recorded, and later transcribed for analysis.
According to the international standard ISO 9241-11, product usability can be evaluated by effectiveness, efficiency, and satisfaction. For evaluating the technical effectiveness, i.e., whether users could successfully complete the tasks, data collected in the pre-trial lab visit were used. The technical effectiveness is calculated by dividing the number of tasks completed successfully by the total number of tasks. The number of errors participants made was also recorded. An error is defined as a mistake such as browsing the wrong app page or an incomplete task. For assessing relative user efficiency, we used relative user efficiency score (RUS), which measures the average time a user took to complete a task in comparison with an expert user of the app [10]. The RUS in this study is the ratio of the time taken to complete a task by participants to that by an expert user, i.e., a study investigator who is part of the development team. In terms of user satisfaction, two self-report instruments including the System Usability Scale (SUS) and the End-user Mobile App Rating Scale (uMARS) are used [11]. Participants completed SUS during the first lab visit and uMARS during the second lab visit. In addition, we assessed user engagement by tracking app usage including frequency of each app page visits and the amount of time spent on each page during the 1-week home trial.
Descriptive statistics were used to summarize the data from all questionnaires, the effectiveness and efficiency test, and the app usage. The qualitative data were collected from semi-structured interviews. All interviews were audio-recorded and transcribed verbatim with pseudonyms for each participant. Content analysis was used to identify and categorize any common trends or themes in the transcripts.
RESULTS
A total of 5 MWUs were recruited and completed the study. All participants were male and aged between 24 and 44 (33.2 ± 8.2) years old. Four of the participants were diagnosed with SCI (including two paraplegia at C5 and unknown level, and two tetraplegia at T2 and T3) and one had spina bifida. Three of the participants already had a habit of exercising regularly. According to the stages of change of PA, three of them were in stage 5 “Maintenance”, one in stage 4 “Decision/Action” and one in stage 3 “Preparation”. Four of them rated their own fitness level as good or excellent, and one rated fair or below. Based on the ESES, they were relatively confident in engaging in regular PA (ESES: 27.8-36.6 out of 40).
During the pre-trial lab visit, after the initial interaction with WheelFit app, 2 participants were very impressed with it, 2 were impressed, and 1 felt somewhat impressed. All five participants expressed interest in tracking push counts and active time, 4 were interested in tracking calories, and 3 were interested in distance traveled. In terms of technical effectiveness, all participants were able to successfully complete the assigned tasks. No major error was recorded during the trial, while two minor errors from two participants (during task “Change the active time goal” and task “Set up a daily exercise reminder with one of the custom workouts in the library”) were recorded. These errors were caused by being confused of what the icons mean as new users of WheelFit. In terms of relative user efficiency, the RUS for each task is shown in Table 1. The participants spent an average of 1.7 times longer than an expert user to complete each task but still completed the tasks within a reasonable time frame.
Tasks assigned to each participant | Time spent on tasks (s) | ||
---|---|---|---|
Participants | Expert | RUS | |
Change the active time goal | 23.4 ± 11.3 | 13.7 | 1.7 ± 0.8 |
Find out the distance traveled 3 days ago | 16.9 ± 3.9 | 8.4 | 2.0 ± 0.5 |
Find out how much calories burnt today at 9 am | 15.0 ± 10.7 | 8.4 | 1.8 ± 1.3 |
Find an aerobic exercise named “Uppercuts” | 21.1 ± 8.4 | 14.5 | 1.5 ± 0.6 |
Set up a daily exercise reminder | 41.7 ± 10.1 | 30.9 | 1.3 ± 0.3 |
Updating the profile with one’s personal information | 46.4 ± 10.8 | 27.4 | 1.7 ± 0.4 |
During the 1-week field trial, user engagement data were collected in an online portal. On average, each participant visited 62.8 ± 28.6 app pages per day and spent 41.6 ± 20.6 minutes per day browsing the app. About 1/3 of the time were spent on checking the home page for a quick overview of their performance. In addition, the progress checking page and workout library were also mostly visited.
After the home-trial, the SUS score of 5 participants were 90, 57.5, 100, 87.5, and 92.5, respectively, with an average of 85.5 ± 16.3. Four participants liked WheelFit a lot and said they would recommend it to their friends. Participant #2 thought the app was okay and had “neutral” responses for a few questions in SUS such as “I found the system unnecessarily complex”, “I thought the system was easy to use”, and “I think I would need the support of a technical person to be able to use this system”. In terms of uMARS score, the composite score was 17.9 ± 1.0 (out of 20), meaning participants thought the app was generally well made. Among the six areas of the uMARS score, WheelFit scored the highest in Information, 19.0 ± 1.2 (out of 20), followed by Aesthetics, 13.4 ± 1.1 (out of 15), Functionality, 17.4 ± 1.7 (out of 20), Engagement, 21.6 ± 2.1 (out of 25), App subjective quality, 14.4 ± 1.5 (out of 20), and Perceived Impact, 21.2 ± 7.5 (out of 30). Participants generally had similar opinions on these six areas except on the perceived impact. Participant #1 rated a total of 8 out of 30 for this area while the rest of the participants rated an average of 24.5 out of 30.
In terms of qualitative feedback, we receive both positive comments as well as suggestions for improvements. Overall participants were impressed with Wheelfit and shared how they used the app.
“I look up exercises I can do in the library that I don’t know already.” – Participant #2
“I use it to check my push counts throughout the day and compare it with how much I normally push so that I know if I am stressing my shoulder too much.” – Participant #3
Participant 1 thought it was a great tool for lots of other MWUs to stay fit, but he personally didn’t think that it was an ideal product for him. He explained that he was a professional athlete and a personal trainer. He knew the importance of having an active lifestyle. He also was very active already and knew all the fitness and health information provided in the app. This explained why he rated “strongly disagree” for statements like “This app has increased my awareness of the importance of having an active lifestyle”, or “This app has changed my attitudes toward more regular physical activity participation” in the uMARS. In general, all 5 participants thought the progress tracking and workout library were the two of the most useful features.
There were also features that participants were not satisfied with. For example, participant 2 pointed out that WheelFit couldn’t seem to detect he was working hard when he did strength training exercises at home. This could be due to the limitation of the custom algorithms not performing well in resistance related activities. Furthermore, all participants thought WheelFit needed improvement in user engagement. Some suggested providing personalized feedback on performance and creating your own workout might improve engagement. In addition, comments that were brought up repeatedly by participants throughout app testing included the Bluetooth disconnection between the smartwatch and the app.
DISCUSSION
Participants in this usability study found the WheelFit app was easy to use and were generally satisfied with the PA measurement and promotion function. According to Bevan’s article on usability measurement, normally novice users need 2 or 3 times longer time to complete a task than an expert user, while in this study it was 1.3 to 2 times longer, this suggests that WheelFit was efficient to use. In this study, the participants were generally satisfied with the app, but some issues and limitations needed to be addressed in the future study. All participants reported having disconnection issue with the smartphone and the Android watch. Participants who used the Huawei Mate 9 Lite phone during the 1-week home trial reported more frequent disconnection than using the Samsung Galaxy S6 phone. This difference suggested that the possible reason for the disconnection might be the system configuration of the manufacture-customized Android system. The small sample size and overall high pre-trial PA level also weakened the external validity of this study. Instead of using their own smartphone, a study smartphone was distributed to each participant. The data collected on the study smartphone may not clearly reflect how they would use the app on their own phone. Since the participants were asked to use the app as much as they could, the user engagement data may not able to represent the real-world scenario. Moreover, it is also very important to develop an IOS version of this app and make more wearable devices accessible to MWUs.
CONCLUSION
The results of this study showed participants were generally satisfied with the functions provided by WheelFit and its overall usability. The instability of the Bluetooth connection between the phone and the Android watch as well as other study limitations such as small sample size were identified. Results of this study could be used as a guide for improving the existing app or developing similar mobile apps for MWUs.
REFERENCE
- LaPlante M, Kaye H. Demographics and trends in wheeled mobility equipment use and accessibility in the community. Assistive Technology. 2010;22(1):3 - 17.
- CDC. Adults with Disabilities -- vital signs for disease control and prevention factsheet 2014. May 6, 2014; http://www.cdc.gov/vitalsigns/disabilities/. Accessed 9/20/2016.
- Hwang, E. J., Groves, M. D., Sanchez, J. N., Hudson, C. E., Jao, R. G., & Kroll, M. E. (2016). Barriers to leisure-time physical activities in individuals with spinal cord injury. Occupational therapy in health care, 30(3), 215-230.
- Schneider, M., Dunton, G. F., & Cooper, D. M. (2008). Physical activity and physical self-concept among sedentary adolescent females: An intervention study. Psychology of sport and exercise, 9(1), 1-14.
- World Health Organization. (2007). Steps to health: A European framework to promote physical activity for health.
- Arbour-Nicitopoulos K, Martin Ginis K, Latimer-Cheung A, Bourne C, Campbell D, Cappe S, Ginis S, Hicks A, Pomerleau P, Smith K. Development of an evidence-informed leisure time physical activity resource for adults with spinal cord injury: the SCI Get Fit Toolkit. Spinal Cord. 2013;51:491 - 500.
- Hiremath, S. V., Amiri, A. M., Thapa-Chhetry, B., Shoaib, N., Snethen, G., Schmidt-Read, M., ... & Intille, S. (2018). Mobile-Health based Physical Activity Intervention for Individuals with Spinal Cord Injury in the Community. Archives of Physical Medicine and Rehabilitation, 99(10), e7.
- Marcus B, Simkin L. The transtheoretical model: applications to exercise behavior Medicine and science in sports and exercise. 1994;26(11):1400-1404.
- Kroll T, Kehn M, Ho P, Groah S. The SCI Exercise Self-Efficacy Scale (ESES): development and psychometric properties. Int J Behav Nutr Phys Act. 2007;4(34).
- Bevan N. Practical Issues in Usability Measurement Interactions. Waits & Measures. 2006.
ACKNOWLEDGMENTS
The authors would like to thank all the participants for sharing their views. This research project was supported by the National Institute of Disability, Independent Living, and Rehabilitation Research (NIDILRR) under Grant #90DP0056-04-00. The content is solely the responsibility of the authors and does not necessarily represent the official views of the NIDILRR.