Samantha R. Schwartz1
, Alyssa J. Schnorenberg
1
, Paige L. Aschenbrener
1
, Lawrence C. Vogel
2
, Brooke A. Slavens
1
1
University of Wisconsin- Milwaukee
2
Shriners Hospitals for Children-Chicago
INTRODUCTION
In the United States, there are approximately 124,000 manual wheelchair users (MWU) under the age of 21 [1], of whom an estimated 60,000 are children with spinal cord injury (SCI). [2, 3] Most studies of MWU with SCI tend to focus on adult populations with very few investigating pediatric wheelchair mobility. [4] Following this lack of pediatric research, current clinical recommendations for wheelchair training have been developed for adults, [5] while none exist specifically for children. Thus, clinicians use the training recommendations designed for adults with pediatric patients, but children are fundamentally different than adults. Children spend a large amount of time in a wheelchair over their lifespan and much musculoskeletal development happens throughout childhood. Additionally, children likely adapt differently to their manual wheelchair, as evidenced by pediatric MWU employing different and more variable propulsion kinematics than adults. [6] Therefore, it is important to consider these differences and to develop wheelchair training recommendations for pediatric populations.
The purpose of this study was to identify changes in handrim kinetics and temporospatial parameters over time in pediatric MWU. These changes may indicate an improvement or worsening in propulsion techniques which could provide insight on when to intervene with interventions such as wheelchair training for injury prevention. The changes may also be connected to the likelihood of developing secondary pain and pathologies including carpal tunnel syndrome, shoulder pain, impingement, and rotator cuff or biceps tendinopathy. By evaluating the changes over time, we can suggest areas of future study to help develop robust and evidence-based training recommendations for pediatric MWU.
METHODS
Participants
Six pediatric MWU with SCI participated in this study during two visits approximately one year apart (Table 1). To be included in this study, participants were required to be under 21 years of age during their first visit and use a manual wheelchair as their primary mode of mobility. The study was approved by university and hospital institutional review boards.
Subject 1 | Subject 2 | Subject 3 | Subject 4 | Subject 5 | Subject 6 | |||||||
---|---|---|---|---|---|---|---|---|---|---|---|---|
Dominant Arm | Right | Right | Right | Right | Right | Right | ||||||
Sex | Female | Male | Male | Male | Male | Male | ||||||
SCI Level / ASIA Score | T11/B | T7/A | T11/A | C6/B | C6/A | C6/C | ||||||
Visit 1 | Visit 2 | Visit 1 | Visit 2 | Visit 1 | Visit 2 | Visit 1 | Visit 2 | Visit 1 | Visit 2 | Visit 1 | Visit 2 |
|
Age (years) | 8.6 | 9.4 | 9.8 | 11.1 | 11.3 | 12.6 | 16.5 | 17.3 | 18.0 | 19.3 | 19.9 | 21.3 |
Time since injury (years) | 3.6 | 4.4 | 1.6 | 3.0 | 1.6 | 3.0 | 0.3 | 1.1 | 0.2 | 1.5 | 2.4 | 3.8 |
Height (cm) | 124 | 135 | 137 | 178 | 154 | 165 | 170 | 170 | 182 | 181 | 178 | 180 |
Weight (kg) | 31.7 | 32.7 | 31.9 | 24.4 | 34.7 | 42.2 | 63.1 | 63.8 | 65.7 | 65.7 | 67.3 | 76.2 |
Data collection
Participants were informed about the testing procedures and assent and parental consent were obtained. Height, weight, and multiple anthropometric measurements of the upper extremities were obtained (e.g., segment circumferences). Twenty-seven passive retro-reflective markers were placed on select anatomical landmarks of the participant using our custom inverse dynamics model. [7] A SmartWheel (Outfront, Pasco, WA) was mounted to the participant's personal wheelchair on the side of their dominant arm. The SmartWheel uninstrumented companion wheel of the same size replaced the participant's wheel on the nondominant side of their wheelchair. Participants propelled their personal manual wheelchair along a 15 meter tile walkway at a self-selected speed using a self-selected stroke pattern. A 14-camera Vicon MX motion capture system using Vicon Nexus software (Vicon Motion Systems, Oxford, UK) collected three-dimensional (3D) kinematics data at 120 Hz while simultaneously, the SmartWheel collected the 3D forces and moments applied by the participant to the handrim at 240 Hz. Multiple trials were collected with adequate rest provided to the participant, as needed.
Data processing
Vicon Nexus software was used to label and fill small gaps in the 3D marker trajectory data and then to filter the data using a Woltring filter with a mean squared error of 20. The 3D kinetic data from the SmartWheel were filtered by a low pass FIR filter and then by an 8th order zero phase Butterworth filter. [8] Each trial was divided into individual wheelchair stroke cycles. The resultant handrim force (FR) was used to identify stroke cycle events via methods outlined by Kwarciak et al. [8] In brief, a stroke cycle began when the participant made contact with the handrim, the start of the contact phase. The contact phase ended when the participant released the handrim, transitioning to the recovery phase. The recovery phase and entire stroke cycle ended right before handrim was made again. To eliminate the effects due to wheelchair acceleration and deceleration, only stroke cycles that occurred during semi-steady-state propulsion were included in the analyses. All usable wheelchair stroke cycles for each participant were analyzed, with multiple stroke cycles per participant per visit.
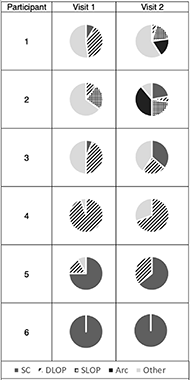
All stroke cycles were then time normalized to percent stroke cycle, with 0% corresponding to the start of the contact phase and 100% as the end of the recovery phase. Custom MATLAB code was used to analyze data from the SmartWheel to calculate key handrim biomechanical metrics within each cycle and create a participant average for each visit. Descriptions and equations for each metric are described in Table 2.
The wheelchair stroke pattern, defined as the motion of the hand during the recovery phase, was determined using the motion of the third metacarpal marker relative to the wheelchair axle in the sagittal plane. The vertical position was plotted against the fore-aft position. [6] Patterns were classified by two independent raters into one of 5 categories: 1) single looping over propulsion (SLOP), 2) double looping over propulsion (DLOP), 3) arcing (Arc), 4) semicircular (SC), and 5) other [5]. The percent use of each stroke pattern for each participant per visit was computed.
RESULTS
All six participants participated in two experimental sessions where Smartwheel and Vicon motion data were collected. The participants spanned different age ranges with no overlap in patient age at time of visit between participants (Table 1).
Changes in the number and frequency of stroke pattern use over time was obtained (Figure 1). The two youngest participants (1 and 2) increased the number of stroke patterns used between visits. The remaining 4 participants primarily used the same number and types of patterns between visits, ranging from only one pattern (participant 6), up to 3 different patterns (participant 3). The oldest participant (6) exclusively used the semicircular pattern type, as recommended for adults, at both visits.
Handrim biomechanical metrics, calculated using Smartwheel data, are presented in Table 2. Wheelchair speed was similar between visits, with a maximum change between visits of only 0.2 m/s in two participants. Length of the contact phase decreased by at least 5% in three participants, increased by at least 5% in two participants, and was relatively unchanged in one participant.
Across subjects, no trends were observed in the change in average peak resultant force per cycle between visits, though participant 4 had a 60% decrease and participant 6 had a substantial increase by 50%. The variance of peak resultant force increased or remained similar in three subjects but dropped in the three oldest participants. In four participants the average maximum rate of rise of the resultant force increased by 45% to 73% between visits.
Metric | Definition | Participant 1 | Participant 2 | Participant 3 | Participant 4 | Participant 5 | Participant 6 | ||||||
---|---|---|---|---|---|---|---|---|---|---|---|---|---|
Visit 1 | Visit 2 | Visit 1 | Visit 2 | Visit 1 | Visit 2 | Visit 1 | Visit 2 | Visit 1 | Visit 2 | Visit 1 | Visit 2 | ||
WC Speed (m/s) | Linear speed of propulsion | 0.9 ± 0.08 | 1.0 ± 0.08 | 1.1 ± 0.05 | 1.3 ± 0.06 | 1.3 ± 0.13 | 1.1 ± 0.08 | 1.1 ± 0.07 | 1.2 ± 0.07 | 1.0 ± 0.07 | 1.0 ± 0.45 | 0.9 ± 0.05 | 1.0 ± 0.06 |
Stroke Frequency (strokes/s) | Number of complete stroke cycles in one second [9] | 0.79 ± 0.07 | 0.81 ± 0.08 | 0.93 ± 0.05 | 1.01 ± 0.05 | 0.85 ± 0.06 | 0.79 ± 0.05 | 0.97 ± 0.05 | 0.99 ± 0.06 | 0.77 ± 0.04 | 0.78 ± 0.35 | 0.77 ± 0.02 | 1.01 ± 0.05 |
Contact End Percentage (%) | Percentage of cycle when contact phase ends [8] | 46.8 ± 3.9 | 37.8 ± 3.7 | 30.5 ± 3.3 | 32.6 ± 4.1 | 26.6 ± 5.9 | 33.3 ± 5.3 | 37.2 ± 3.2 | 32.2 ± 4.6 | 53.2 ± 3.7 | 39.8 ± 16.5 | 41.1 ± 4.4 | 52.5 ± 3.7 |
Peak FR (N) | Maximum resultant force [10] | 22.4 ± 3.6 | 32.5 ± 4.5 | 23.0 ± 5.3 | 29.0 ± 8.5 | 26.3 ± 12.7 | 27.1 ± 4.8 | 55.4 ± 6.7 | 22.6 ± 5.0 | 48.8 ± 3.5 | 45.4 ± 21.4 | 52.0± 8.8 | 79.8 ± 14.8 |
Variance of Fres | Coefficient of variance= mean Fres/SD [11] | 6.62 | 7.79 | 5.32 | 5.36 | 2.98 | 6.79 | 7.58 | 3.58 | 11.5 | 2.13 | 6.18 | 5.53 |
Max Rate of Rise of Fres (N/s) | Maximum value of derivative of Fres [10] | 559.4 ± 248 | 917.8 ± 245 | 882.7 ± 364 | 1283 ± 702 | 689.7 ± 299 | 620.0 ± 189 | 2744 ± 694 | 420.2 ± 65 | 1436 ± 449 | 2480 ± 1488 | 1095 ± 408 | 1816 ± 576 |
DISCUSSION
Knowledge about pediatric manual wheelchair use and its effects on users over time is lacking in current literature. This study investigated handrim biomechanics, temporal parameters, and stroke pattern use in six pediatric MWU at two visits over time, approximately one year apart. This is the first quantitative longitudinal assessment of pediatric wheelchair biomechanics. Results showed changes in stroke patterns, kinetics, and temporal parameters between visits. Finding are noteworthy, as some of these changes have been associated with pain and pathology in adults.
The hand pattern during the recovery period of the stroke cycle (i.e., stroke pattern) has been extensively studied in adults in relation to propulsion efficiency and overuse injuries due to the repetitive nature of propulsion. [5] Specifically, recommendations are to use a semicircular hand pattern, to decrease stroke frequency and to increase time spent in the contact phase relative to recovery phase. [5, 6] Our results further support that of our previous work that found pediatric MWU used unique and highly variable stroke patterns compared to adults. [4] Interestingly, between visits all but the oldest participant changed the frequency with which they used certain propulsion patterns, but only two increased the use of the semicircular pattern. This variability in stroke patterns may be related to the lower incidence of shoulder pain in MWU with pediatric-onset SCI compared to adults. [12] Between visits three participants decreased their time spent in the contact phase, which opposes what is recommended for adults.
Previous research in adults has related specific handrim kinetic metrics with clinical presentation of upper extremity pain and pathology. Specifically, decreased median nerve function (a precursor for carpal tunnel syndrome) has been directly associated with increases in the rate of rise of resultant force, the resultant force, and the stroke frequency. [13] This study found that a majority of the pediatric participants exhibited an increase in the rate of rise of resultant force, the peak resultant force, and stroke frequency at the second visit. Additionally, decreased variability in peak resultant force of the hand-rim is related to increased shoulder pain [14] and repetitive strain injuries in MWU. We found that half of the participants exhibited increased variability in peak resultant force at the handrim, while the other half exhibited decreased variability in peak resultant force. These findings may suggest an increased risk of developing carpal tunnel syndrome and shoulder pain over time in pediatric MWU without appropriate and timely pediatric-based interventions. This work warrants further investigation to determine optimal surveillance methods to prevent pathological developments in pediatric MWU.
CONCLUSIONS
This study successfully quantified changes over a year's timespan in clinically relevant handrim metrics for a group of pediatric MWU. Many of these changes have been associated with upper limb pain and pathology in adults. These findings support the use of further longitudinal analyses to help develop interventions and pediatric guidelines to improve wheelchair propulsion and protect the upper extremities over the lifespan of MWU.
REFERENCES
- Brault W. Americans With Disabilities: 2010 Household Economic Studies Current Population Reports. 2012.
- National Spinal Cord Injury Statistical Center, Spinal Cord Injury Facts and Figures at a Glance. Birmingham, AL: University of Alabama-Birmingham, 2020.
- Facts on Pediatric Spinal Cord Injury. American Spinal Injury Association; 2020.
- Slavens BA, Schnorenberg AJ, Aurit CM, Tarima S, Vogel LC, Harris GF. Biomechanics of Pediatric Manual Wheelchair Mobility. Frontiers in Bioengineering and Biotechnology. 2015;3:137.
- Preservation of Upper Limb Function Following Spinal Cord Injury: A Clinical Practice Guideline for Health-Care Professionals. Consortium for Spinal Cord Medicine; 2005.
- Boninger ML, Souza AL, Cooper RA, Fitzgerald SG, Koontz AM, Fay BT. Propulsion patterns and pushrim biomechanics in manual wheelchair propulsion. Archives of physical medicine and rehabilitation. 2002;83(5):718-23.
- Schnorenberg AJ, Slavens BA, Wang M, Vogel LC, Smith PA, Harris GF. Biomechanical model for evaluation of pediatric upper extremity joint dynamics during wheelchair mobility. Journal of biomechanics. 2014;47(1):269-76.
- Kwarciak AM, Sisto SA, Yarossi M, Price R, Komaroff E, Boninger ML. Redefining the manual wheelchair stroke cycle: identification and impact of nonpropulsive pushrim contact. Archives of physical medicine and rehabilitation. 2009;90(1):20-6.
- Schottler J, Graf A, Kelly E, Vogel L. Training Youth With SCI to Improve Efficiency and Biomechanics of Wheelchair Propulsion: A Pilot Study. Top Spinal Cord Inj Rehabil. 2019;25(2):157-63.
- Bossuyt FM, Hogaboom NS, Worobey LA, Koontz AM, Arnet U, Boninger ML. Start-up propulsion biomechanics changes with fatiguing activity in persons with spinal cord injury. J Spinal Cord Med. 2019:1-9.
- Moon Y, Jayaraman C, Hsu I, Rice I, Hsiao-Wecksler E, Sosnoff J. Variability of peak shoulder force during wheelchair propulsion in manual wheelchair users with and without shoulder pain. Clinical Biomechanics. 2013;28(9-10):967-72.
- Brose SW, Boninger ML, Fullerton B, McCann T, Collinger JL, Impink BG, et al. Shoulder ultrasound abnormalities, physical examination findings, and pain in manual wheelchair users with spinal cord injury. Archives of physical medicine and rehabilitation. 2008;89(11):2086-93.
- Boninger ML, Cooper RA, Baldwin MA, Shimada SD, Koontz A. Wheelchair pushrim kinetics: body weight and median nerve function. Archives of physical medicine and rehabilitation. 1999;80(8):910-5.
- Rice IM, Jayaraman C, Hsiao-Wecksler ET, Sosnoff JJ. Relationship between shoulder pain and kinetic and temporal-spatial variability in wheelchair users. Archives of physical medicine and rehabilitation. 2014;95(4):699-704.
ACKNOWLEDGEMENTS
This research was supported by the Eunice Kennedy Shriver National Institute Of Child Health & Human Development of the National Institutes of Health under award 1R01HD098698 and the National Institute on Disability, Independent Living, and Rehabilitation Research grant number 90RE5006-01-00.